Artificial intelligence and its task-specific subset, machine learning, are transforming many fields. In ATAP, these tools are being developed and applied to stabilize laser and electron beams; find optimal operating points in complex multivariate parameter spaces; detect and classify signs of a coming “quench,” or loss of superconductivity, in a high-field magnet; and anticipate and steer clear of operational problems. The overarching goal: machines and beams that better serve the needs of users in discovery science and applications.
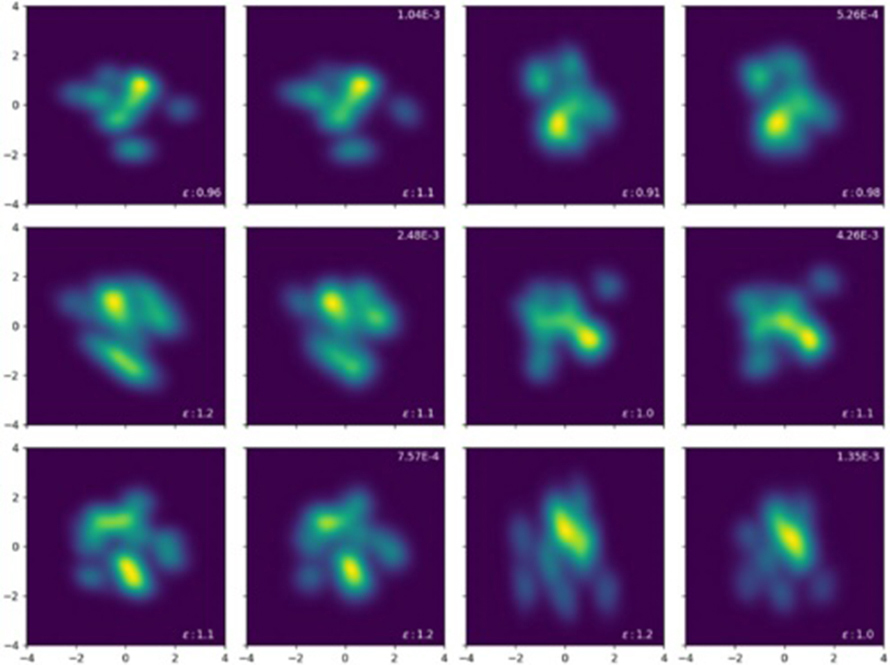
AMP researches and applies multifidelity Bayesian optimization, trains neural network surrogates with high-performance simulations, explores AI/ML guided strategies for lattice design, couples AI/ML with high-performance supercomputing and experiments toward “smart superfacilities,” and leads a course on AI/ML for particle accelerators at the US Particle Accelerator School.
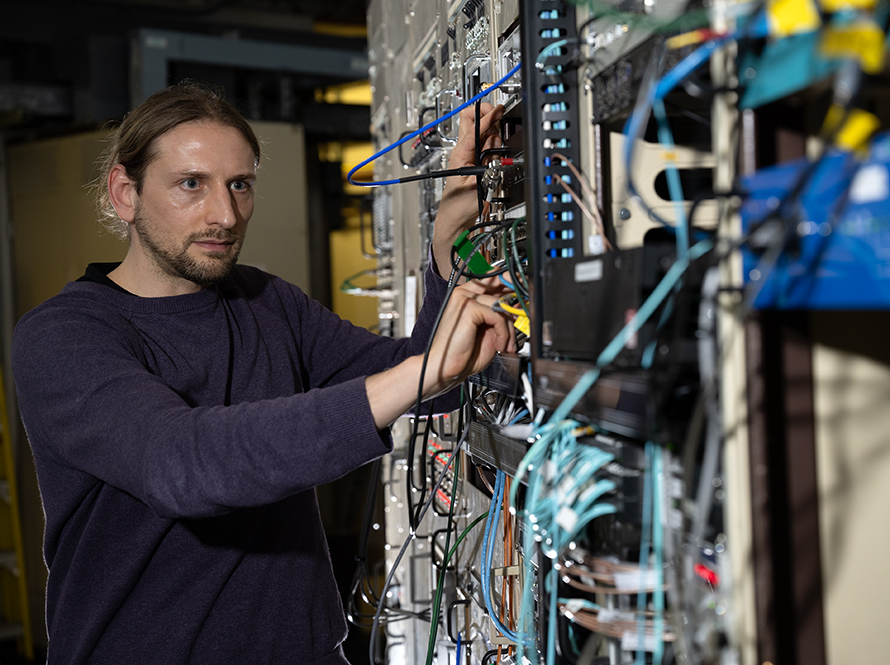
ATAP’s Advanced Light Source Accelerator Physics Program studies and applies AI/ML techniques for improving performance of existing accelerators, as well as for designing future accelerators like the ALS Upgrade and improving their operation. We pride ourselves on having been the first group in the world to deploy ML-based optimization on a synchrotron light source during routine user operations.

The advanced particle accelerators of tomorrow will require equally advanced controls and instrumentation, being developed by ATAP’s Berkeley Accelerator Controls and Instrumentation (BACI) Program. BACI also develops Innovative fiber laser technologies for laser-plasma accelerators. AI/ML is bringing new levels of performance to both those realms.
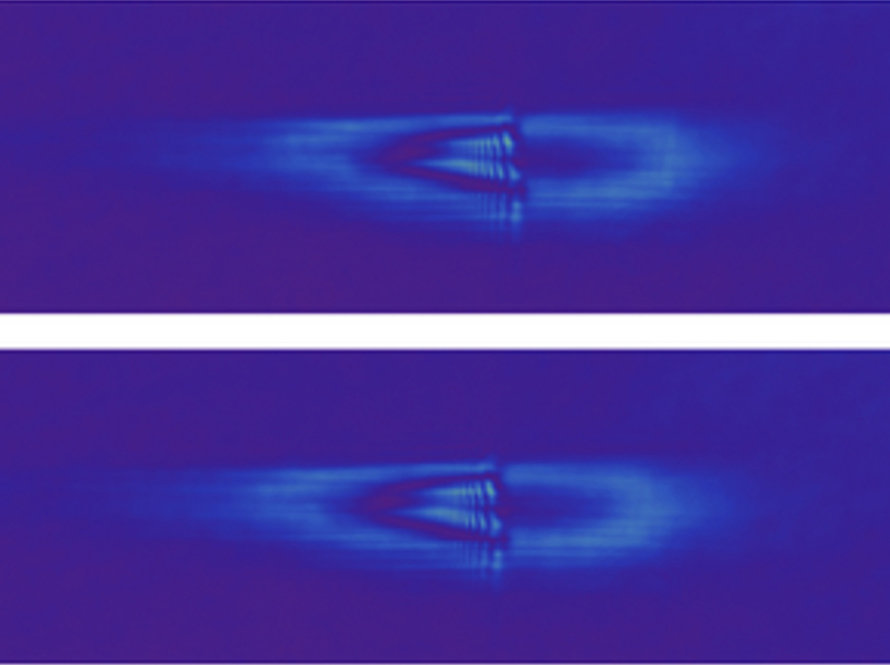
The Berkeley Lab Laser Accelerator (BELLA) Center is integrating AI/ML into the operation, optimization, and diagnosis of the laser-plasma accelerator and its light source derivatives. Rapid data analysis, driving active stabilization components, and mapping out key correlations between laser, plasma, and particle beam parameters, are key areas where AI/ML has strong impact.

Small local losses of superconductivity can propagate and render a magnet normally-conductive. This “quench” requires immediate measures to handle the stored energy of the magnet and also the high-powered particle beam that it guides. Our Superconducting Magnet Program (SMP) is applying ML to detect early signs of an upcoming quench. They also use automated AI/ML analysis of microscopy images to better understand and optimize commercial superconductor.